Observação
Clique aqui para baixar o código de exemplo completo
Criando mapas de calor anotados #
Muitas vezes, é desejável mostrar os dados que dependem de duas variáveis independentes como um gráfico de imagem com código de cores. Isso geralmente é chamado de mapa de calor. Se os dados forem categóricos, isso seria chamado de mapa de calor categórico.
A função do Matplotlib imshow
torna a produção de tais gráficos particularmente fácil.
Os exemplos a seguir mostram como criar um mapa de calor com anotações. Vamos começar com um exemplo fácil e expandi-lo para ser utilizável como uma função universal.
Um simples mapa de calor categórico #
Podemos começar definindo alguns dados. O que precisamos é de uma lista ou array 2D que defina os dados para o código de cores. Também precisamos de duas listas ou matrizes de categorias; é claro que o número de elementos nessas listas precisa corresponder aos dados ao longo dos respectivos eixos. O mapa de calor em si é um imshow
gráfico com os rótulos definidos para as categorias que temos. Observe que é importante definir os locais dos marcadores ( ) e set_xticks
também os rótulos dos marcadores ( set_xticklabels
), caso contrário, eles ficarão fora de sincronia. Os locais são apenas os números inteiros ascendentes, enquanto os marcadores são os rótulos a serem exibidos. Por fim, podemos rotular os próprios dados criando um Text
dentro de cada célula mostrando o valor dessa célula.
import numpy as np
import matplotlib
import matplotlib as mpl
import matplotlib.pyplot as plt
vegetables = ["cucumber", "tomato", "lettuce", "asparagus",
"potato", "wheat", "barley"]
farmers = ["Farmer Joe", "Upland Bros.", "Smith Gardening",
"Agrifun", "Organiculture", "BioGoods Ltd.", "Cornylee Corp."]
harvest = np.array([[0.8, 2.4, 2.5, 3.9, 0.0, 4.0, 0.0],
[2.4, 0.0, 4.0, 1.0, 2.7, 0.0, 0.0],
[1.1, 2.4, 0.8, 4.3, 1.9, 4.4, 0.0],
[0.6, 0.0, 0.3, 0.0, 3.1, 0.0, 0.0],
[0.7, 1.7, 0.6, 2.6, 2.2, 6.2, 0.0],
[1.3, 1.2, 0.0, 0.0, 0.0, 3.2, 5.1],
[0.1, 2.0, 0.0, 1.4, 0.0, 1.9, 6.3]])
fig, ax = plt.subplots()
im = ax.imshow(harvest)
# Show all ticks and label them with the respective list entries
ax.set_xticks(np.arange(len(farmers)), labels=farmers)
ax.set_yticks(np.arange(len(vegetables)), labels=vegetables)
# Rotate the tick labels and set their alignment.
plt.setp(ax.get_xticklabels(), rotation=45, ha="right",
rotation_mode="anchor")
# Loop over data dimensions and create text annotations.
for i in range(len(vegetables)):
for j in range(len(farmers)):
text = ax.text(j, i, harvest[i, j],
ha="center", va="center", color="w")
ax.set_title("Harvest of local farmers (in tons/year)")
fig.tight_layout()
plt.show()
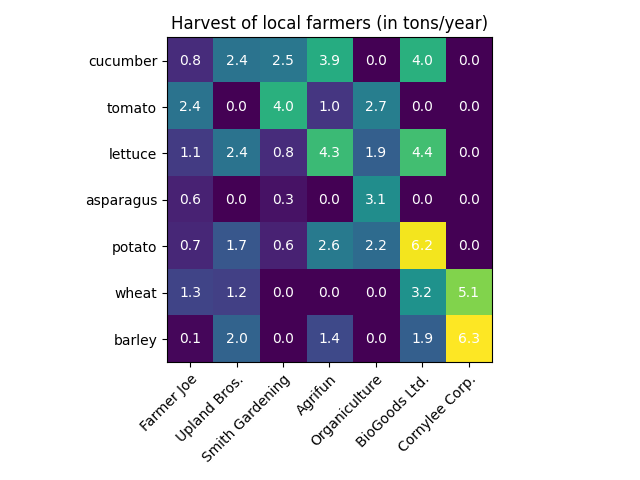
Usando o estilo de código de função auxiliar #
Conforme discutido nos estilos de codificação, pode-se querer reutilizar esse código para criar algum tipo de mapa de calor para diferentes dados de entrada e/ou em diferentes eixos. Criamos uma função que recebe os dados e os rótulos de linha e coluna como entrada e permite argumentos que são usados para personalizar o gráfico
Aqui, além do acima, também queremos criar uma barra de cores e posicionar os rótulos acima do mapa de calor em vez de abaixo dele. As anotações devem ter cores diferentes dependendo de um limite para melhor contraste com a cor do pixel. Por fim, desativamos os espinhos dos eixos circundantes e criamos uma grade de linhas brancas para separar as células.
def heatmap(data, row_labels, col_labels, ax=None,
cbar_kw=None, cbarlabel="", **kwargs):
"""
Create a heatmap from a numpy array and two lists of labels.
Parameters
----------
data
A 2D numpy array of shape (M, N).
row_labels
A list or array of length M with the labels for the rows.
col_labels
A list or array of length N with the labels for the columns.
ax
A `matplotlib.axes.Axes` instance to which the heatmap is plotted. If
not provided, use current axes or create a new one. Optional.
cbar_kw
A dictionary with arguments to `matplotlib.Figure.colorbar`. Optional.
cbarlabel
The label for the colorbar. Optional.
**kwargs
All other arguments are forwarded to `imshow`.
"""
if ax is None:
ax = plt.gca()
if cbar_kw is None:
cbar_kw = {}
# Plot the heatmap
im = ax.imshow(data, **kwargs)
# Create colorbar
cbar = ax.figure.colorbar(im, ax=ax, **cbar_kw)
cbar.ax.set_ylabel(cbarlabel, rotation=-90, va="bottom")
# Show all ticks and label them with the respective list entries.
ax.set_xticks(np.arange(data.shape[1]), labels=col_labels)
ax.set_yticks(np.arange(data.shape[0]), labels=row_labels)
# Let the horizontal axes labeling appear on top.
ax.tick_params(top=True, bottom=False,
labeltop=True, labelbottom=False)
# Rotate the tick labels and set their alignment.
plt.setp(ax.get_xticklabels(), rotation=-30, ha="right",
rotation_mode="anchor")
# Turn spines off and create white grid.
ax.spines[:].set_visible(False)
ax.set_xticks(np.arange(data.shape[1]+1)-.5, minor=True)
ax.set_yticks(np.arange(data.shape[0]+1)-.5, minor=True)
ax.grid(which="minor", color="w", linestyle='-', linewidth=3)
ax.tick_params(which="minor", bottom=False, left=False)
return im, cbar
def annotate_heatmap(im, data=None, valfmt="{x:.2f}",
textcolors=("black", "white"),
threshold=None, **textkw):
"""
A function to annotate a heatmap.
Parameters
----------
im
The AxesImage to be labeled.
data
Data used to annotate. If None, the image's data is used. Optional.
valfmt
The format of the annotations inside the heatmap. This should either
use the string format method, e.g. "$ {x:.2f}", or be a
`matplotlib.ticker.Formatter`. Optional.
textcolors
A pair of colors. The first is used for values below a threshold,
the second for those above. Optional.
threshold
Value in data units according to which the colors from textcolors are
applied. If None (the default) uses the middle of the colormap as
separation. Optional.
**kwargs
All other arguments are forwarded to each call to `text` used to create
the text labels.
"""
if not isinstance(data, (list, np.ndarray)):
data = im.get_array()
# Normalize the threshold to the images color range.
if threshold is not None:
threshold = im.norm(threshold)
else:
threshold = im.norm(data.max())/2.
# Set default alignment to center, but allow it to be
# overwritten by textkw.
kw = dict(horizontalalignment="center",
verticalalignment="center")
kw.update(textkw)
# Get the formatter in case a string is supplied
if isinstance(valfmt, str):
valfmt = matplotlib.ticker.StrMethodFormatter(valfmt)
# Loop over the data and create a `Text` for each "pixel".
# Change the text's color depending on the data.
texts = []
for i in range(data.shape[0]):
for j in range(data.shape[1]):
kw.update(color=textcolors[int(im.norm(data[i, j]) > threshold)])
text = im.axes.text(j, i, valfmt(data[i, j], None), **kw)
texts.append(text)
return texts
O exposto acima agora nos permite manter a criação do enredo real bastante compacta.
fig, ax = plt.subplots()
im, cbar = heatmap(harvest, vegetables, farmers, ax=ax,
cmap="YlGn", cbarlabel="harvest [t/year]")
texts = annotate_heatmap(im, valfmt="{x:.1f} t")
fig.tight_layout()
plt.show()
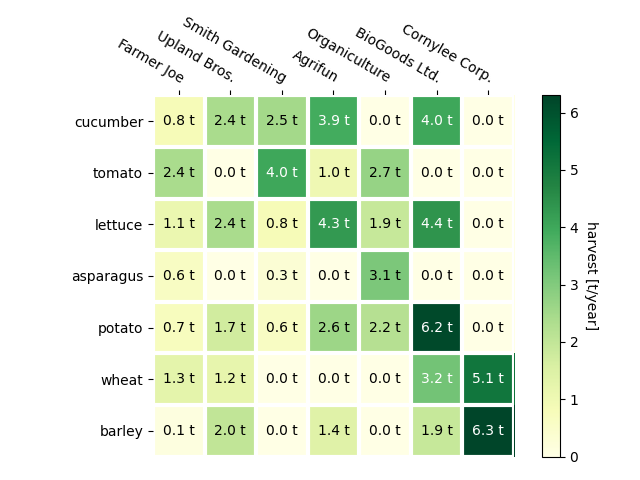
Alguns exemplos de mapas de calor mais complexos #
A seguir, mostramos a versatilidade das funções criadas anteriormente, aplicando-as em diferentes casos e usando diferentes argumentos.
np.random.seed(19680801)
fig, ((ax, ax2), (ax3, ax4)) = plt.subplots(2, 2, figsize=(8, 6))
# Replicate the above example with a different font size and colormap.
im, _ = heatmap(harvest, vegetables, farmers, ax=ax,
cmap="Wistia", cbarlabel="harvest [t/year]")
annotate_heatmap(im, valfmt="{x:.1f}", size=7)
# Create some new data, give further arguments to imshow (vmin),
# use an integer format on the annotations and provide some colors.
data = np.random.randint(2, 100, size=(7, 7))
y = ["Book {}".format(i) for i in range(1, 8)]
x = ["Store {}".format(i) for i in list("ABCDEFG")]
im, _ = heatmap(data, y, x, ax=ax2, vmin=0,
cmap="magma_r", cbarlabel="weekly sold copies")
annotate_heatmap(im, valfmt="{x:d}", size=7, threshold=20,
textcolors=("red", "white"))
# Sometimes even the data itself is categorical. Here we use a
# `matplotlib.colors.BoundaryNorm` to get the data into classes
# and use this to colorize the plot, but also to obtain the class
# labels from an array of classes.
data = np.random.randn(6, 6)
y = ["Prod. {}".format(i) for i in range(10, 70, 10)]
x = ["Cycle {}".format(i) for i in range(1, 7)]
qrates = list("ABCDEFG")
norm = matplotlib.colors.BoundaryNorm(np.linspace(-3.5, 3.5, 8), 7)
fmt = matplotlib.ticker.FuncFormatter(lambda x, pos: qrates[::-1][norm(x)])
im, _ = heatmap(data, y, x, ax=ax3,
cmap=mpl.colormaps["PiYG"].resampled(7), norm=norm,
cbar_kw=dict(ticks=np.arange(-3, 4), format=fmt),
cbarlabel="Quality Rating")
annotate_heatmap(im, valfmt=fmt, size=9, fontweight="bold", threshold=-1,
textcolors=("red", "black"))
# We can nicely plot a correlation matrix. Since this is bound by -1 and 1,
# we use those as vmin and vmax. We may also remove leading zeros and hide
# the diagonal elements (which are all 1) by using a
# `matplotlib.ticker.FuncFormatter`.
corr_matrix = np.corrcoef(harvest)
im, _ = heatmap(corr_matrix, vegetables, vegetables, ax=ax4,
cmap="PuOr", vmin=-1, vmax=1,
cbarlabel="correlation coeff.")
def func(x, pos):
return "{:.2f}".format(x).replace("0.", ".").replace("1.00", "")
annotate_heatmap(im, valfmt=matplotlib.ticker.FuncFormatter(func), size=7)
plt.tight_layout()
plt.show()
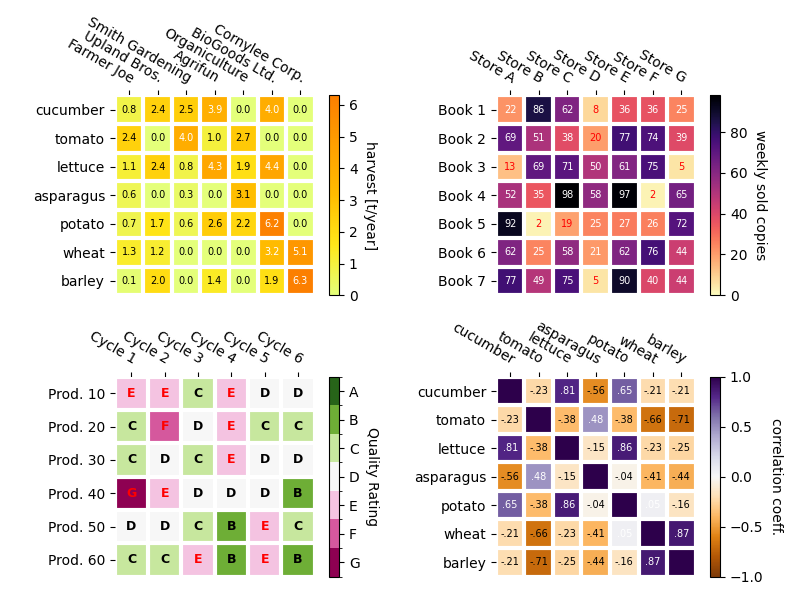
Referências
O uso das seguintes funções, métodos, classes e módulos é mostrado neste exemplo:
Tempo total de execução do script: ( 0 minutos 2,652 segundos)